The Ultimate Guide to Video Label Tools for Data Annotation
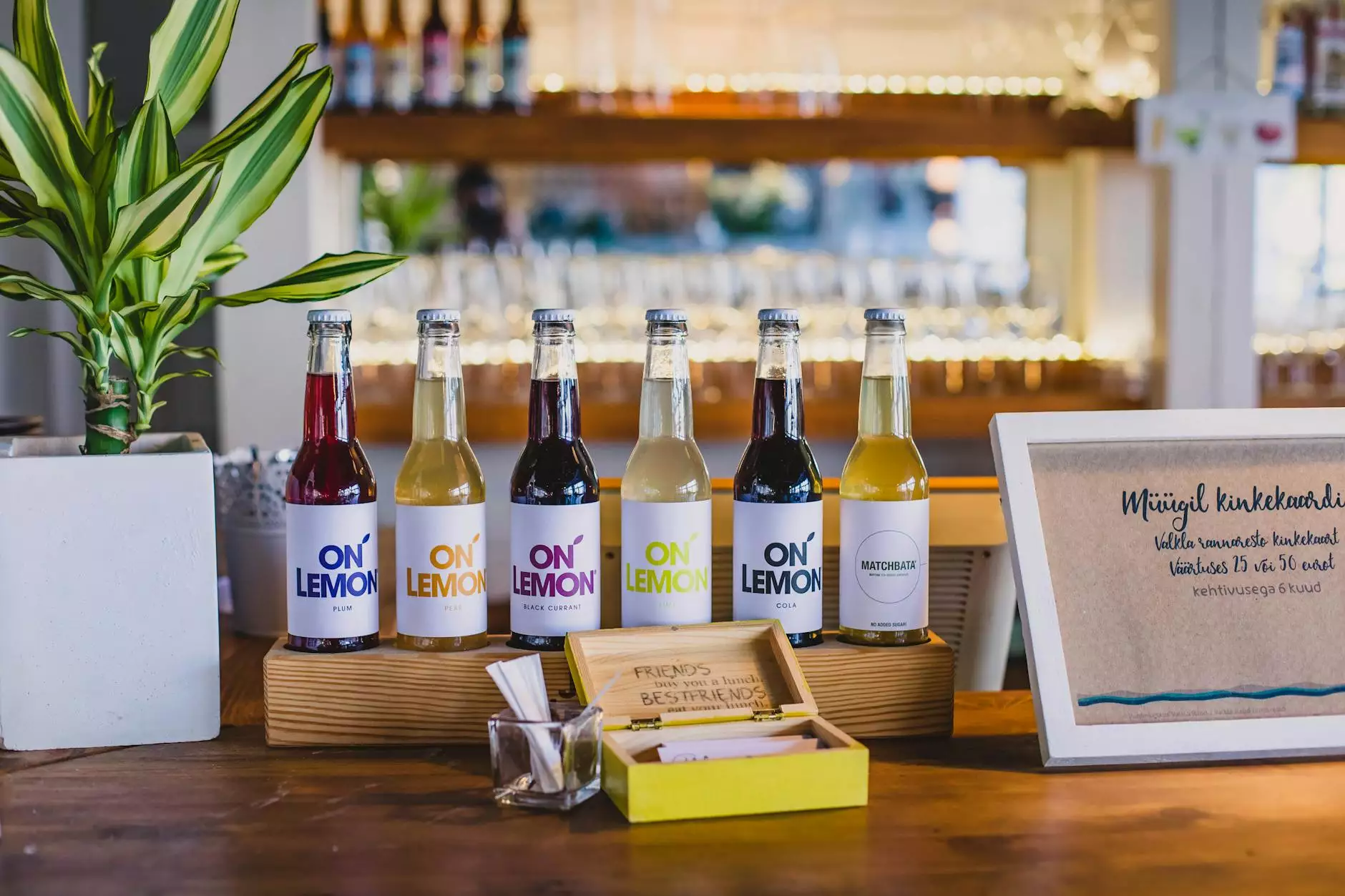
In today's data-driven world, businesses and researchers are increasingly relying on advanced technologies to improve their decision-making processes. One such critical technology is the video label tool, which plays a pivotal role in the realm of data annotation. At KeyLabs.ai, we understand the importance of high-quality data annotation in enhancing machine learning models and overall business efficiency. This article explores the significance of video label tools and provides comprehensive insights for businesses seeking to leverage them effectively.
Understanding Video Label Tools
A video label tool is a specialized software application designed to facilitate the annotation of video data. This tool enables users to label objects, actions, and other relevant features within video frames, thereby creating structured datasets that are essential for training machine learning algorithms. The efficiency of a video label tool significantly influences the accuracy of the machine learning models that rely on the annotated data.
Key Features of Video Label Tools
When selecting a video label tool, it's essential to consider several key features:
- Intuitive User Interface: A user-friendly interface enhances productivity and minimizes the learning curve for new users.
- Multi-format Support: The tool should support various video formats to accommodate different data sources.
- Collaborative Features: Team collaboration functionalities allow multiple users to work on annotations simultaneously.
- Advanced Annotation Techniques: Features such as bounding boxes, segmentation, and keypoint annotations provide comprehensive data tagging.
- Export Options: Flexible export options ensure that the labeled data can be seamlessly integrated into machine learning pipelines.
- AI-assisted Labeling: Utilizing AI to automate parts of the labeling process can significantly speed up data preparation.
Why Businesses Should Invest in Video Labeling Tools
Investing in a video label tool offers numerous benefits for businesses, particularly those involving AI and machine learning:
1. Enhanced Data Quality
Quality data is the foundation of any successful machine learning project. A video label tool ensures precise labeling, which directly correlates with improved model performance and better decision-making outcomes.
2. Increased Productivity
Automated and semi-automated labeling processes reduce the time required for data annotation, allowing teams to focus on other critical tasks, thus enhancing overall productivity.
3. Cost Efficiency
Despite an initial investment, the efficiency gained through streamlined processes and improved data quality leads to significant long-term cost savings.
4. Scalability
As the demand for labeled data grows, a robust video label tool can adapt to expanding projects, enabling businesses to scale their operations effectively.
5. Competitive Advantage
Organizations that leverage advanced video labeling tools can develop superior machine learning applications, thereby gaining a significant edge over competitors who rely on traditional data annotation methods.
Best Practices for Using Video Label Tools
To maximize the benefits of a video label tool, businesses should adhere to the following best practices:
1. Define Annotation Guidelines
Establish clear and concise guidelines for video labeling. These guidelines should include the types of annotations required and the specific criteria for each label, ensuring consistency across the team.
2. Train Your Team
Invest in training your team on how to use the video label tool effectively. Familiarity with its features maximizes productivity and minimizes errors.
3. Leverage Collaboration
Encourage collaboration among team members using the tool's shared capabilities. This approach fosters communication and enhances the quality of annotations through collective feedback.
4. Monitor Progress
Regularly review the annotation process to ensure adherence to guidelines and to identify any issues promptly. Monitoring helps maintain the quality and efficiency of the annotation workflow.
5. Continuously Improve
Solicit feedback from users and continuously refine your annotation processes. Staying agile and open to improvement fosters innovation and efficiency in data labeling tasks.
Challenges in Video Annotation
While video labeling tools offer immense benefits, businesses may face several challenges when incorporating them into their data workflows:
1. Complexity of Video Data
Videos are inherently complex, often containing varying elements such as multiple objects, scenes, and movements. This complexity necessitates advanced tools that can handle diverse annotation requirements.
2. Time-Consuming Process
Even with labeling tools, annotating video data can still be quite time-consuming, particularly for lengthy videos or those with intricate details. Integrating AI-assisted features can help alleviate this issue.
3. Annotation Consistency
Ensuring that all annotators follow established guidelines can be challenging, especially in larger teams. Continuous training and feedback loops can help maintain consistency.
4. Data Privacy Concerns
When dealing with sensitive video content, businesses must navigate data privacy laws and regulations. Implementing strict data protection protocols is essential to address these concerns.
Conclusion: The Future of Video Labeling with KeyLabs.ai
As the demand for high-quality annotated data continues to rise, the role of the video label tool becomes increasingly vital. At KeyLabs.ai, we are committed to providing state-of-the-art data annotation tools that cater to the diverse needs of businesses today and in the future. Embracing video labeling technology not only enhances your machine learning capabilities but also positions your business for success in a competitive landscape.
Investing in a robust video label tool will empower your teams to produce high-quality datasets, leading to greater insights and better machine learning models. As we move towards a more automated and data-centric future, businesses that prioritize effective data annotation will undoubtedly stay ahead of the curve.